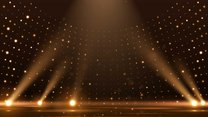
In the future, many companies will rely on artificial intelligence (AI) to optimize their business processes; for example, in the logistics to detect potential transport delays at an early stage and counteract these delays. The predictions are based on AI models that are trained to predict the course of business processes using large amounts of data. This works very well, as numerous works in research and practice demonstrate. However, the use of AI models has one major drawback: the prediction results of the AI models are not readily understandable or comprehensible – neither for normal users nor for AI experts. The prediction models are so complex that they must be regarded as black box.
Prof. Pohl’s research group is investigating technologies that can be used to explain the results of the black-box methods. Specifically for AI-generated business process predictions, the paluno team has developed the counterfactual method LORELEY. This method breaks down which specific characteristics of the business process data are responsible for the prediction. From this, explanations can be derived that not only make predications more understandable, but at the same time recommend actions. They show how better prediction results could be achieved by changing the characteristics. For example, in the case of predicted delivery delays, logisticians can use this information to make informed decisions about how to adjust processes to avoid or at least to mitigate the delays.
Generation of Interpretable Models
The paluno scientists deduce the counterfactual explanations from interpretable decision tree models (see Figure). LORELEY trains the decision trees with samples from the complex AI model so that the decision trees (for these samples) produce similar prediction results as the original AI model. Experiments with real-world datasets show that the LORELEY approach can approximate the results of the complex prediction models very accurately, i.e., with a fidelity of up to 98%. “The induced explanations can help to increase user confidence in AI applications,” says Prof. Pohl. “At the same time, they help experts to better understand AI models and their underlying training data and respond appropriately to predictions.”
Current Publication
Tsung-Hao Huang, Andreas Metzger and Klaus Pohl: Counterfactual Explanations for Predictive Business Process Monitoring. In: Marinos Themistocleous and Maria Papadaki (eds.): Information Systems - 18th European, Mediterranean, and Middle Eastern Conference, EMCIS 2021, Virtual Event, December 8-9, 2021, Proceedings , Volume 437 of Lecture Notes in Business Information Processing , Springer , 2021 , 399-413. [DOI]
Contact
Software Systems Engineering (SSE) | +49 201 18-34660 klaus.pohl@paluno.uni-due.de |
Press and Public Relations | +49 201 18-34655 birgit.kremer@paluno.uni-due.de |